Decode the Algorithm: Navigate the World of Machine Learning in Business with 'The AI Playbook'
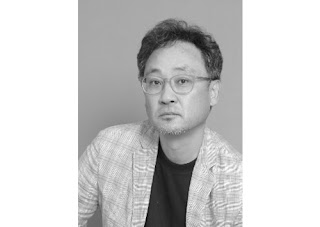
Yong Xune Xon PhD. Revision Consulting. Seoul Korea. xyxonxyxon@empal.com
"Machine Learning Times" published https://www.predictiveanalyticsworld.com/machinelearningtimes/decode-the-algorithm-navigate-the-world-of-machine-learning-in-business-with-the-ai-%E2%80%8B%E2%80%8Bplaybook/13365/
In my 30 years of studying, researching, and applying machine learning models for prediction and anomaly detection in various business environments, I've consistently felt that there are too few people who understand why they are trying to implement this technology. Whether they are data scientists (now often referred to as data analysts), users or managers who need to use the results of machine learning, or executives who approve the projects, almost all have little to no understanding of what they are creating, why they are creating it, or what results ML should deliver.
'The AI Playbook' is a book about the application of machine learning in real organizations, calmly explaining many very important and fundamental aspects that most data scientists, machine learning engineers, managers, and various practitioners – in other words, the ultimate users of machine learning – do not know or overlook.

https://www.amazon.com/AI-Playbook-Mastering-Deployment-Management/dp/0262048906
Among the many precious stories this book discusses, let's examine a few. Elevating human understanding and leadership is more crucial for the success of machine learning than the technology itself. When business leaders are presented with a model as the outcome of an enthusiastically initiated project, they often reject its deployment. This rejection stems not only from technical limitations but also from a lack of understanding of ML's value within the organization.
The first step of ML is to define the goal. This involves defining the business value proposition and deciding how ML will improve the task (when deployed). However, less than half of the decision-makers in organizations understand data and the stories conveyed through visualization and models. If this issue is not addressed, ML can become meaningless, a waste of resources, or worse, a source of misinformation causing problems within the organization.
I believe that the CRISP-DM, introduced in the 1990s, was a groundbreaking work for its time, offering innovative ideas and well-defined procedures. It was significant because it added a step to the then prevalent methodology of statistical analysis and machine learning, which was technology-centric, to concretize the business objectives and expected outcomes, thereby clarifying why organizations use technology.
However, it's regrettable that even after a considerable time, practices for applying ML in business have not become standardized or universally adopted. 'The AI Playbook' is built on the foundation of bizML, which is the culmination of the author's extensive practical experience aimed at complementing and expanding CRISP-DM.
The philosophy of bizML is that in business, one should focus on the final decision-making and work backwards from there. It emphasizes the need to first define and start with how the business aspect should be changed through the technology of ML. I completely agree that setting the goals of ML deployment through reverse planning can keep the project on the right track. Analysts who have experienced both failures and successes in ML intuitively or instinctively work in this manner, even if they do not directly learn and apply such specific frameworks or methodologies. This is because it is a natural flow for successful ML project execution.
The AI Playbook' meticulously explains how the ML process should be implemented in business, using concrete real-life examples such as UPS's package prediction. Moreover, the book provides crucial details such as when predictions should be obtained for them to hold business value and what the real business implication of what is commonly referred to as 'accuracy' is.
Recently, the spotlight seems to be solely on generative AI like ChatGPT. However, generative AI is also a part of machine learning and shares many potential pitfalls outlined in this book. Therefore, the discussions in this book are essential for those only interested in generative AI.

(Image DALL-E3 in ChatGPT. prompt :: A two-shot image contrasting two scenes: 1) An AI robot lost and wandering in a desert, looking confused and aimless amidst vast sand dunes under a scorching sun, and 2) the same AI robot working productively in an office, efficiently handling multiple tasks with focus and precision, surrounded by computers, files, and office equipment. The image should be in a vibrant, maximalistic pop art style, emphasizing bright, bold colors and dynamic compositions. The shape of the image should be wide.)
This book will be immensely helpful for data scientists and machine learning engineers in developing and deploying truly useful machine learning models more effectively in practice. It will also serve as a significant guide for business leaders, managers, and operational staff who aspire to successfully implement machine learning in business, helping them understand the value of machine learning and the necessary actions to take. Furthermore, for end-users of machine learning models, this book will aid in understanding how the models are developed and how their outputs should be interpreted and utilized. Even if the book feels somewhat challenging, I believe that perusing just a third or half of its contents will be undoubtedly beneficial.
[ 한글 번역 ]
"알고리즘을 해독하라: 'The AI Playbook'으로 비즈니스에서의 머신러닝 세계를 항해하라"
30년 간 다양한 비즈니스 환경에서 예측과 이상 탐지를 위한 기계 학습 모델을 연구하고 적용하면서, 기술을 도입하려는 사람들 중 이해하는 사람이 너무 적다는 것을 느꼈다. 데이터 과학자(현재는 데이터 분석가로 종종 언급됨), 기계 학습의 결과를 사용해야 하는 사용자나 관리자, 프로젝트를 승인하는 경영진까지 거의 모두 자신들이 만들고 있는 것과 그 이유, ML이 제공해야 할 결과에 대해 거의 이해하지 못한다.
'The AI Playbook'은 실제 조직에서 기계 학습을 적용하는 것에 대한 책으로, 많은 중요하고 기본적인 측면을 차분하게 설명한다. 이는 대부분의 데이터 과학자, 기계 학습 엔지니어, 관리자 및 다양한 실무자들 - 즉, 기계 학습의 최종 사용자들이 모르거나 간과하는 것들이다.
이 책에서 다루는 많은 귀중한 이야기 중 몇 가지를 살펴보면, 기술 자체보다 인간의 이해와 리더십이 기계 학습의 성공에 더 중요하다. 비즈니스 리더들이 열정적으로 시작된 프로젝트의 결과로 모델을 제시받았을 때, 종종 그 배포를 거부한다. 이 거부는 기술적 한계뿐만 아니라 조직 내 ML의 가치에 대한 이해 부족에서 비롯된다.
ML의 첫 단계는 목표를 정의하는 것이다. 이는 비즈니스 가치 제안을 정의하고 ML이 배포될 때 작업을 어떻게 개선할 것인지 결정하는 것을 포함한다. 그러나 조직의 의사 결정자 중 절반 미만이 데이터와 시각화 및 모델을 통해 전달되는 이야기를 이해한다. 이 문제가 해결되지 않으면 ML은 의미가 없게 되거나, 자원 낭비, 또는 조직 내 문제를 야기하는 오정보의 원인이 될 수 있다.
1990년대에 도입된 CRISP-DM은 당시 혁신적인 아이디어와 잘 정의된 절차를 제공한 혁신적인 작업이었다. 기술 중심이었던 당시의 통계 분석 및 기계 학습 방법론에 비즈니스 목표와 예상 결과를 구체화하는 단계를 추가했기 때문에 중요했다.
그러나 상당한 시간이 지났음에도 불구하고, 비즈니스에서 ML을 적용하는 관행이 표준화되거나 보편적으로 채택되지 않은 것은 아쉽다. 'The AI Playbook'은 저자의 광범위한 실무 경험을 바탕으로 한 bizML의 기반 위에 구축되었다
bizML의 철학은 비즈니스에서 최종 결정에 초점을 맞추고, 거기에서부터 역으로 ML 기술을 통해 비즈니스 측면을 어떻게 변화시킬지 정의하고 시작하는 것이다. ML 배포의 목표를 역으로 계획하는 것이 프로젝트를 올바른 방향으로 유지하는 데 도움이 될 수 있다고 전적으로 동의한다. ML에서 실패와 성공을 경험한 분석가들은 이러한 특정 프레임워크나 방법론을 직접 배우고 적용하지 않더라도, 직관적으로나 본능적으로 이런 방식으로 작업한다. 이는 성공적인 ML 프로젝트 실행을 위한 자연스러운 흐름이기 때문이다.
'The AI Playbook'은 UPS의 패키지 예측과 같은 구체적인 실제 사례를 사용하여 비즈니스에서 ML 프로세스를 어떻게 구현해야 하는지 자세히 설명한다. 또한, 예측이 비즈니스 가치를 가질 수 있는 시점과 일반적으로 '정확도'라고 언급되는 것의 실제 비즈니스 의미와 같은 중요한 세부 사항을 제공한다.
최근 ChatGPT와 같은 생성적 AI에 대한 관심이 집중되고 있지만, 생성적 AI도 기계 학습의 일부이며 이 책에서 다룬 많은 잠재적 함정을 공유한다. 따라서 이 책의 논의는 생성적 AI에만 관심이 있는 사람들에게도 필수적이다.
이 책은 데이터 과학자와 기계 학습 엔지니어가 실제로 유용한 기계 학습 모델을 더 효과적으로 개발하고 배포하는 데 큰 도움이 될 것이다. 또한 비즈니스에서 기계 학습을 성공적으로 구현하고자 하는 비즈니스 리더, 관리자 및 운영 직원에게 중요한 안내서 역할을 하며, 그들이 기계 학습의 가치와 필요한 조치를 이해하는 데 도움이 될 것이다. 더 나아가 기계 학습 모델의 최종 사용자에게는 모델이 어떻게 개발되고 그 출력이 어떻게 해석되고 활용되어야 하는지 이해하는 데 도움이 될 것이다. 책이 다소 어렵게 느껴질 수 있지만, 내용의 삼분의 일이나 절반만 읽어도 분명히 유익할 것이다.
'인공지능' 카테고리의 다른 글
CES 2024, 생성AI 전쟁. 그리고 생성AI 경쟁에서의 승자의 조건 (1) | 2024.01.13 |
---|---|
[promptStrategies TIPs Series #5] ChatGPT 검색 기능으로 시장 조사하기: 5 Pitfalls (0) | 2024.01.11 |
전략이란 무엇인가 (feat. ChatGPT) (0) | 2024.01.08 |
2024 기업들의 생성AI 경쟁 전략: Critical Edges (0) | 2024.01.06 |
경영학도의 "디지털 마케터의 챗GPT 활용 전략" 독파 계획서 24 (1) | 2024.01.05 |